Artificial intelligence (AI) has advanced rapidly in recent years, with systems capable of generating text, moderating content, and even producing art. However, the power of AI also comes with risks, as these systems could potentially be misused to create spam, fake content, or perpetrate fraud.
To combat this, technology companies have developed an AI detector—a system that analyzes text, images, videos and other content to determine whether it was produced by a human or an AI algorithm. These detectors open up several beneficial use cases across industries.
Academic Integrity
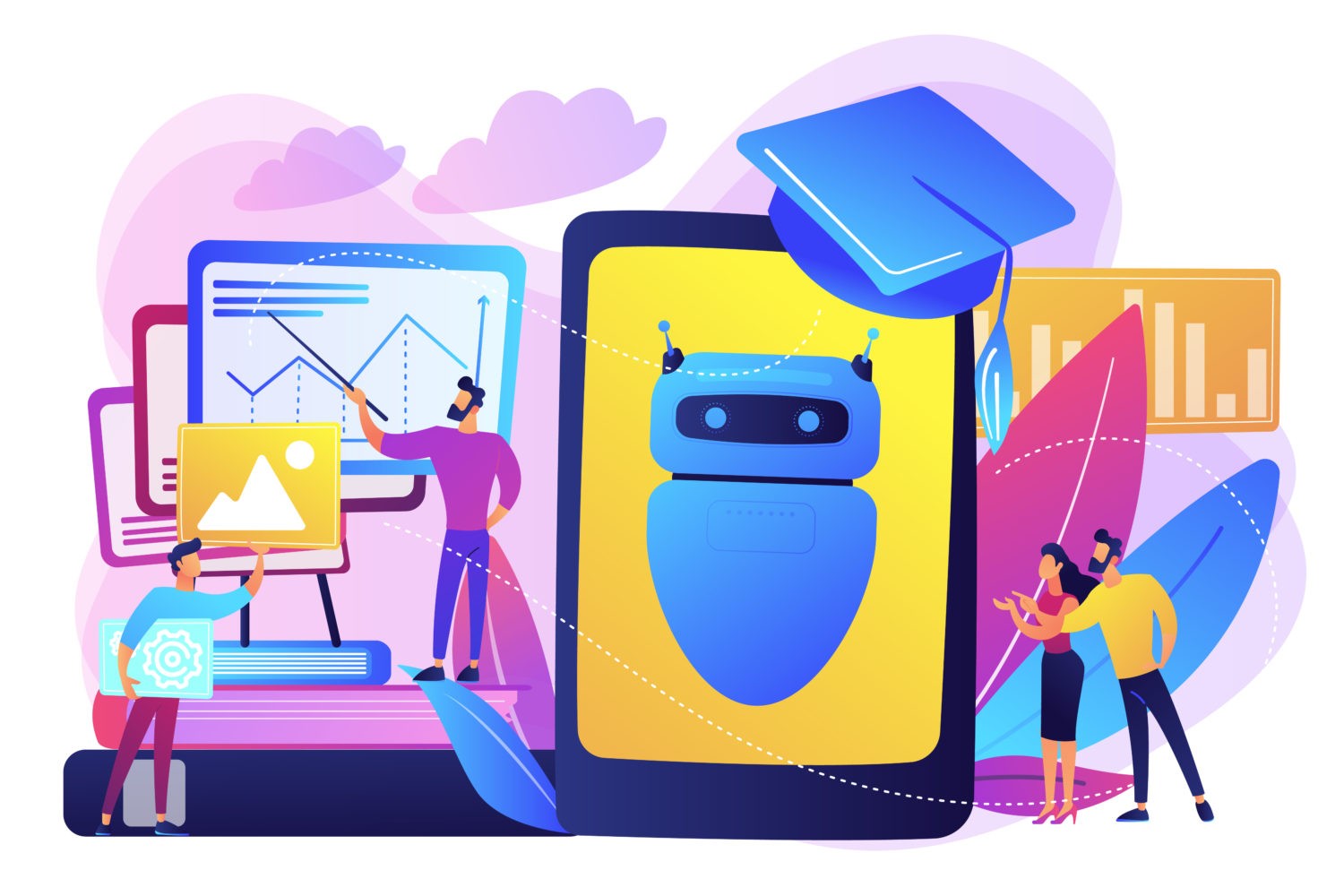
Maintaining academic integrity is crucial for education quality. However, cheating and plagiarism undermine this integrity. Studies suggest over 60% of college students have engaged in some form of cheating.
AI detectors can analyze student submissions like essays to identify writing quality issues, stylistic inconsistencies and plagiarized passages. This allows instructors to uphold academic standards.
Specific use cases include:
Essay Scoring
AI scoring systems can evaluate factors like thesis clarity, evidence quality, formatting and grammar to assign essay scores. This relieves instructors’ grading workload so they can focus on providing qualitative feedback.
The algorithmic scores also offer standardized benchmarking across large classes. Automated scoring correlation with human graders ranges from 0.75 to 0.95 depending on the essay criteria.
Plagiarism Detection
AI detectors compare student submissions against current and archived online content to identify similarity percentages and potential source material. Many systems also recognize commonly plagiarized phrases and paper mill content.
Schools use these detectors to scan assignments before grading. Students can also use plagiarism checkers on their work to avoid accidental copying. These tools make it harder for students to pass off others’ writing as their own.
Authorship Verification
Stylometry algorithms analyze writing style features like vocabulary, syntax, and formatting. This allows determining whether an assignment matches a student’s usual writing style.
Schools use verification to confirm students completed their own work. The AI assesses style consistency across a student’s submissions, flagging larger divergences for further inspection.
While not definitive evidence of cheating, improbable style deviations prompt deeper investigation by academics. Authorship verification makes it riskier for students to succeed by having others complete their assignments.
Content Moderation
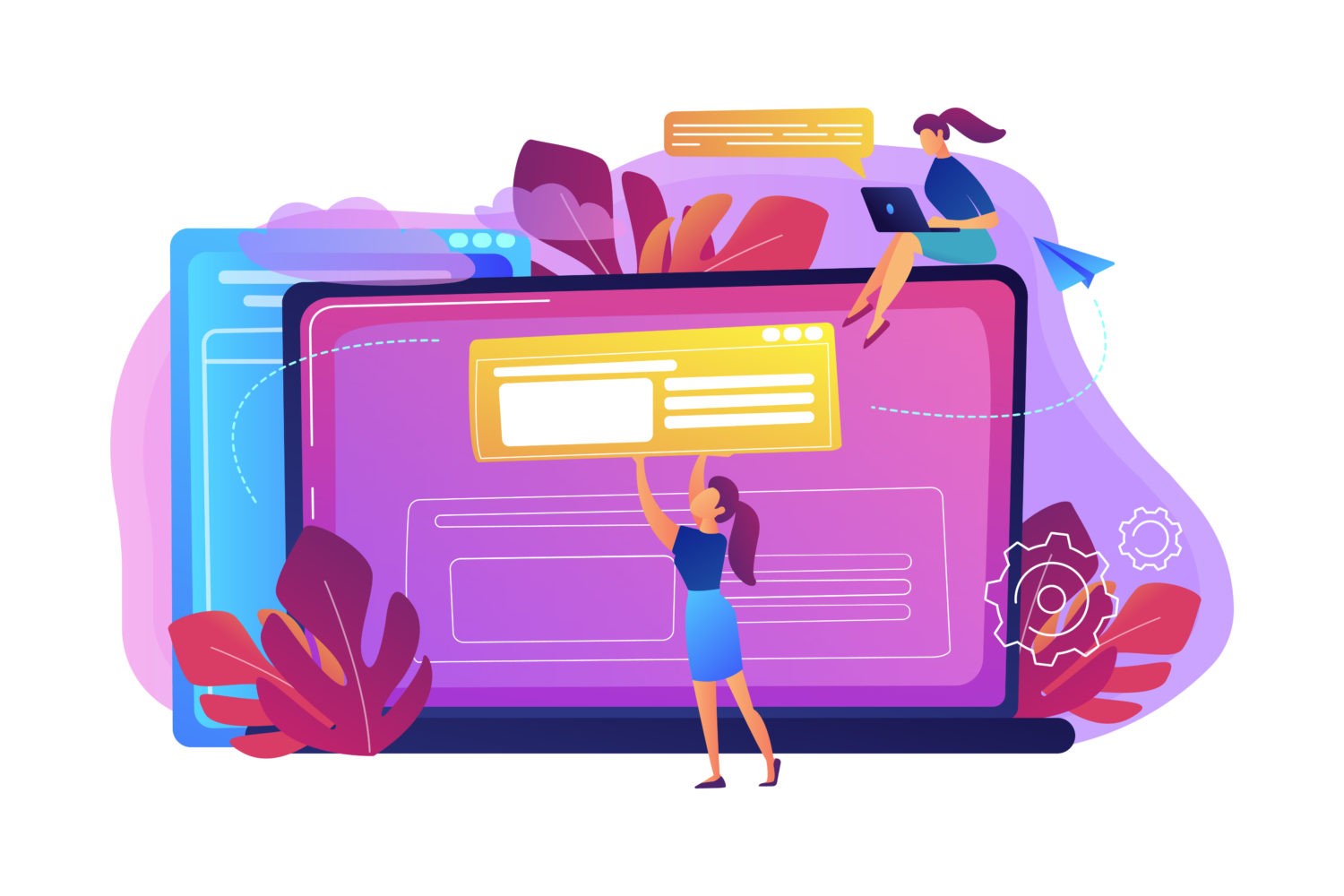
User-generated content platforms need scalable and accurate content control to provide quality experiences. AI detectors give websites, social networks and gaming communities advanced moderation capabilities.
Text Moderation
AI text analysis searches user posts for policy violations like profanity, harassment, hate speech and misinformation based on language patterns. It automatically flags or hides inappropriate content for human review.
Text moderation is essential for community quality and brand safety. It allows faster response to toxic comments that drive away users. The combination of algorithmic flagging and human judgement balances enforcement and speech concerns.
YouTube, Facebook, Twitter and Reddit all use AI text analysis among wider content control toolsets. These detectors also enable customization to address moderation gaps around marginalized group abuse.
Image and Video Moderation
Visual content requires different detection approaches focused on object, scene and activity recognition. AI scans images and video for policy breaches around nudity, violence or dangerous behavior using machine vision techniques.
This allows digital platforms to block prohibited visual material, like extremism and child exploitation imagery. Automated flagging facilitates human inspection at scale for final take down decisions.
Some systems can also identify manipulated “deepfake” media. This limits misinformation spread and reputational damage from high-profile face swaps and political hoaxes.
Policy Compliance Audits
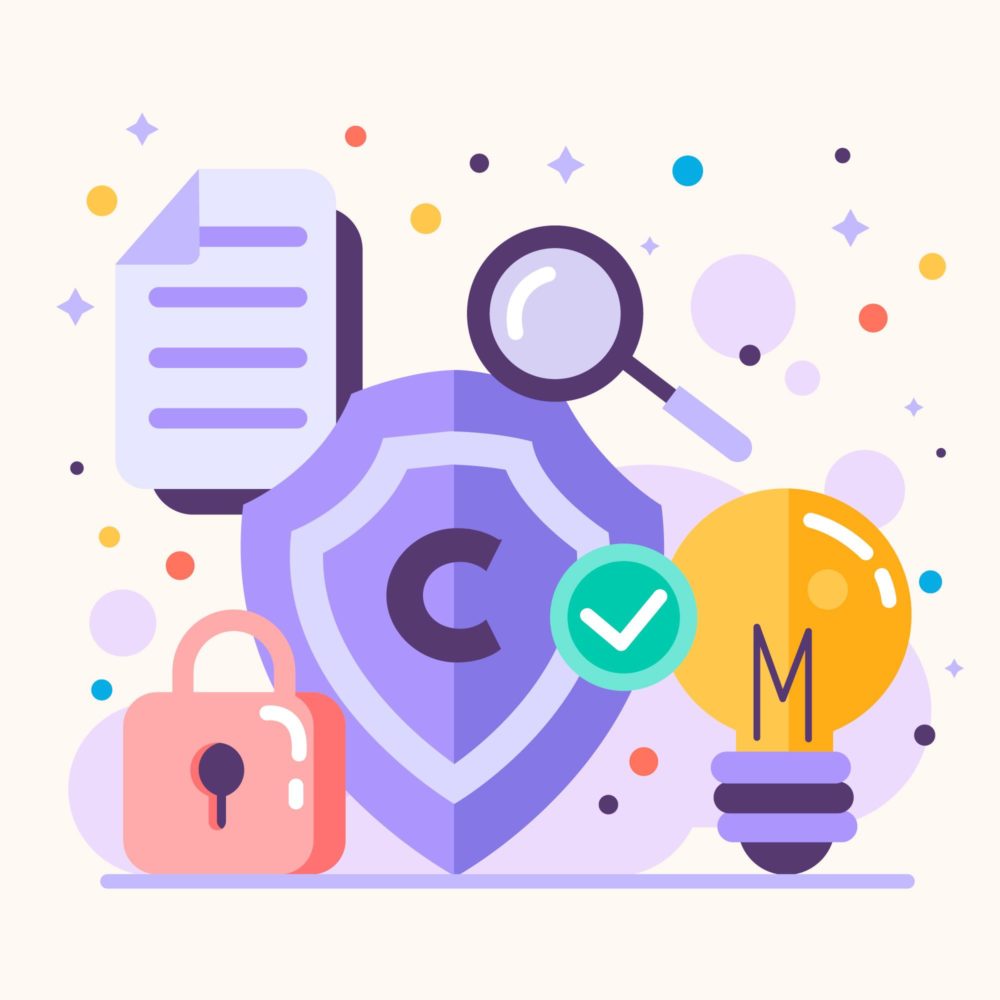
Moderation AI can run platform audits analyzing historic information flows around target issues like vaccine misinformation or state-linked influence operations. These audits retrospectively trace policy violations and exposure to assist remediation.
Network mapping visualizations from audits give transparency into past platform spread patterns. Companies leverage the audit insights to update detection rules and community guidelines addressing identified issues.
Fraud Detection
Fraudulent activities like spam, phishing and inauthentic account networks undermine platform trust. AI analyzes account traits, behaviors and connections to catch policy violators.
Detector algorithms feed suspicious signals to site integrity teams for review. Fraud rate estimations inform resource allocation and site improvements to counter malicious activities.
Responsible AI Concerns
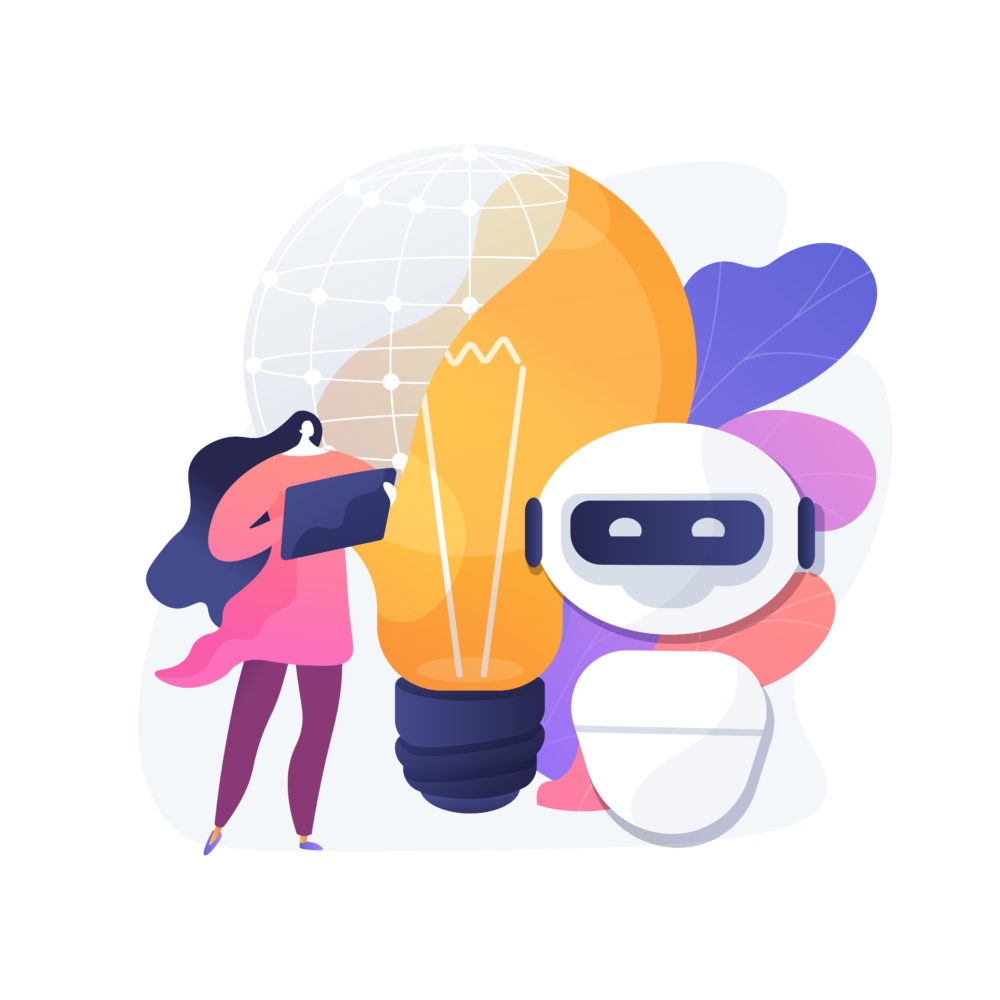
Advancing accountability around AI detectors will maximize benefits while navigating risks like accuracy biases, ethical issues and malicious use cases.
Algorithmic Bias and Fairness
Dataset biases and subjective design choices can lead detector algorithms to unfairly target marginalized demographic groups or misclassify benign content.
Ongoing algorithm audits, upgraded training processes and human-machine moderation combinations help mitigate accuracy and equity issues. Still, detectors should undergo continuous bias testing before and after launch.
Transparency
Clearly communicating detection system capabilities, limitations and error rates builds understanding for why specific moderation actions occur. This transparency tempers unfair expectations while highlighting areas needing performance improvement.
However, excessive technical detail could enable malicious actors to game detectors. Balancing transparency aims against security risks around AI vulnerabilities remains an open challenge.
Misuse Risks
Powerful detection abilities also lend themselves to harmful applications like mass surveillance, predictive policing and censorship automation contrary to norms of accountability and human rights.
Governance frameworks, ethics reviews and self-regulation practices should guide the development of integrity use cases over regimes of social control. But ongoing vigilance around misuse will stay necessary as capabilities expand.
Outlook
AI detectors supply scalable integrity capabilities for managing knowledge creation, communications and transactions. Our reliance on algorithmic analysis for these functions will only increase as content volumes grow.
Yet detectors merely operationalize underlying social priorities around issues like academic excellence, community norms and fraud prevention. Improving detectors means improving our collective ability to articulate and govern for these social goods.
Through collaboration and ethical innovation, AI can progress both integrity and inclusion goals vital for human flourishing. But achieving this future depends on how we choose to deploy and direct today’s detection systems.
FAQ
How accurate are AI detectors?
Accuracy varies by use case but averages over 90%. Teams continuously update algorithms on new data to improve precision.
What content do detectors analyze?
Detectors scan text, images, video, audio and metadata. Different data types require tailored AI models.
Can detectors be fooled?
Systems have vulnerabilities to adversarial inputs designed to mislead them. Companies try minimizing these integrity gaps through security testing.
Do algorithms replace human moderators?
No, AI and humans complement each other. Automated signals focus reviewer resources for better decisions.
⸻ Author Bio ⸻
Jane Doe is an AI professional with several years of experience in the technology field. She works at InnovateTech Solutions, where she develops AI systems for content moderation and fraud detection. Jane is dedicated to creating fair and transparent AI technologies and frequently writes about these topics for various publications.